The use of generative AI tools (e.g. ChatGPT) in education is now common among young people (see data from the UK’s Ofcom regulator). As a computing educator or researcher, you might wonder what impact generative AI tools will have on how young people learn programming. In our latest research seminar, Barbara Ericson and Xinying Hou (University of Michigan) shared insights into this topic. They presented recent studies with university student participants on using generative AI tools based on large language models (LLMs) during programming tasks.
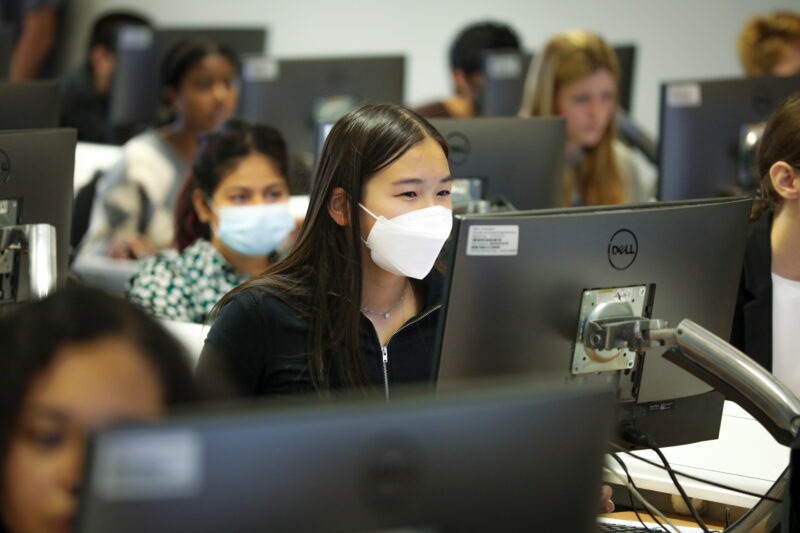
Using Parson’s Problems to scaffold student code-writing tasks
Barbara and Xinying started their seminar with an overview of their earlier research into using Parson’s Problems to scaffold university students as they learn to program. Parson’s Problems (PPs) are a type of code completion problem where learners are given all the correct code to solve the coding task, but the individual lines are broken up into blocks and shown in the wrong order (Parsons and Haden, 2006). Distractor blocks, which are incorrect versions of some or all of the lines of code (i.e. versions with syntax or semantic errors), can also be included. This means to solve a PP, learners need to select the correct blocks as well as place them in the correct order.
In one study, the research team asked whether PPs could support university students who are struggling to complete write-code tasks. In the tasks, the 11 study participants had the option to generate a PP when they encountered a challenge trying to write code from scratch, in order to help them arrive at the complete code solution. The PPs acted as scaffolding for participants who got stuck trying to write code. Solutions used in the generated PPs were derived from past student solutions collected during previous university courses. The study had promising results: participants said the PPs were helpful in completing the write-code problems, and 6 participants stated that the PPs lowered the difficulty of the problem and speeded up the problem-solving process, reducing their debugging time. Additionally, participants said that the PPs prompted them to think more deeply.
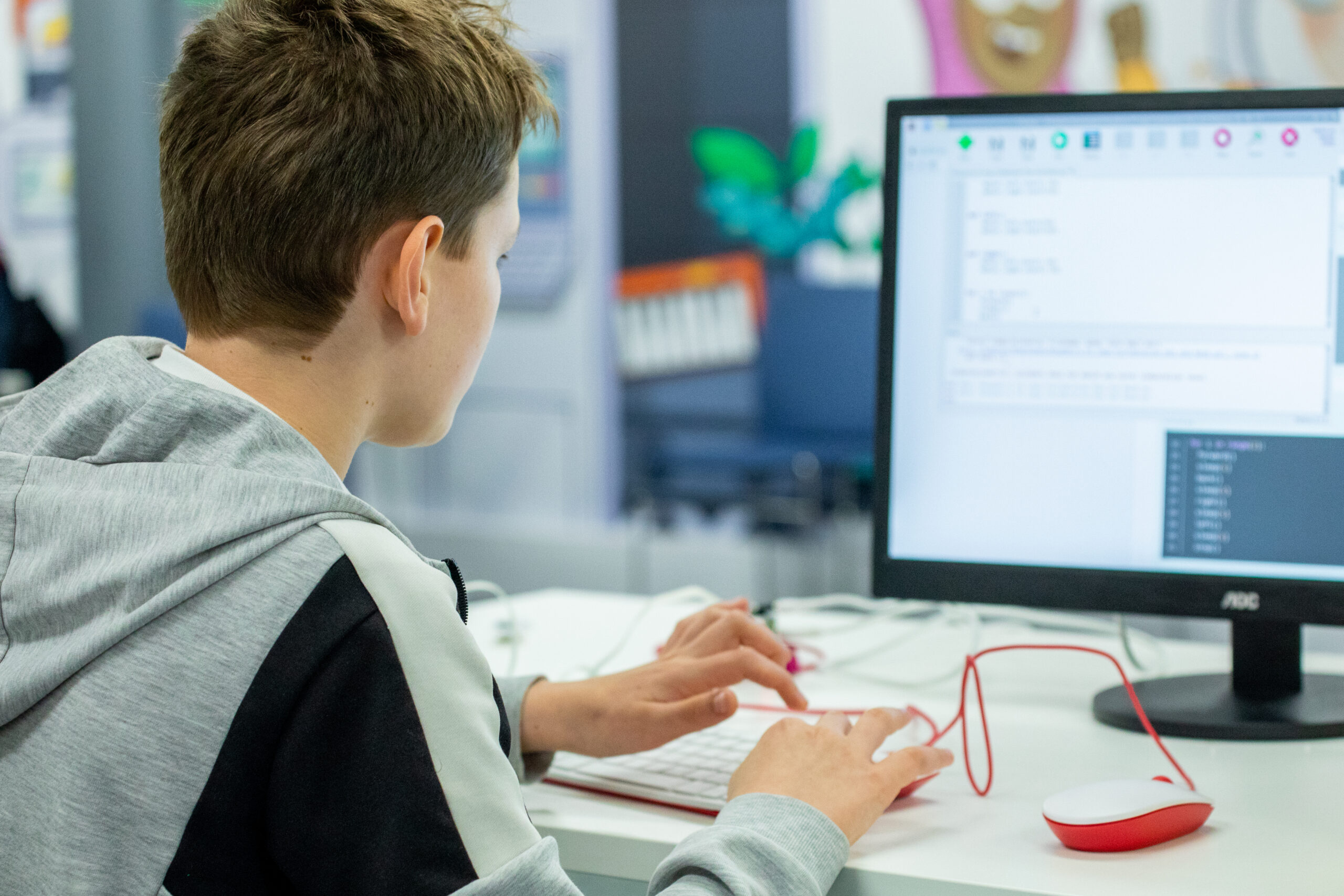
This study provided further evidence that PPs can be useful in supporting students and keeping them engaged when writing code. However, some participants still had difficulty arriving at the correct code solution, even when prompted with a PP as support. The research team thinks that a possible reason for this could be that only one solution was given to the PP, the same one for all participants. Therefore, participants with a different approach in mind would likely have experienced a higher cognitive demand and would not have found that particular PP useful.
Supporting students with varying self-efficacy using PPs
To understand the impact of using PPs with different learners, the team then undertook a follow-up study asking whether PPs could specifically support students with lower computer science self-efficacy. The results show that study participants with low self-efficacy who were scaffolded with PPs support showed significantly higher practice performance and higher problem-solving efficiency compared to participants who had no scaffolding. These findings provide evidence that PPs can create a more supportive environment, particularly for students who have lower self-efficacy or difficulty solving code writing problems. Another finding was that participants with low self-efficacy were more likely to completely solve the PPs, whereas participants with higher self-efficacy only scanned or partly solved the PPs, indicating that scaffolding in the form of PPs may be redundant for some students.
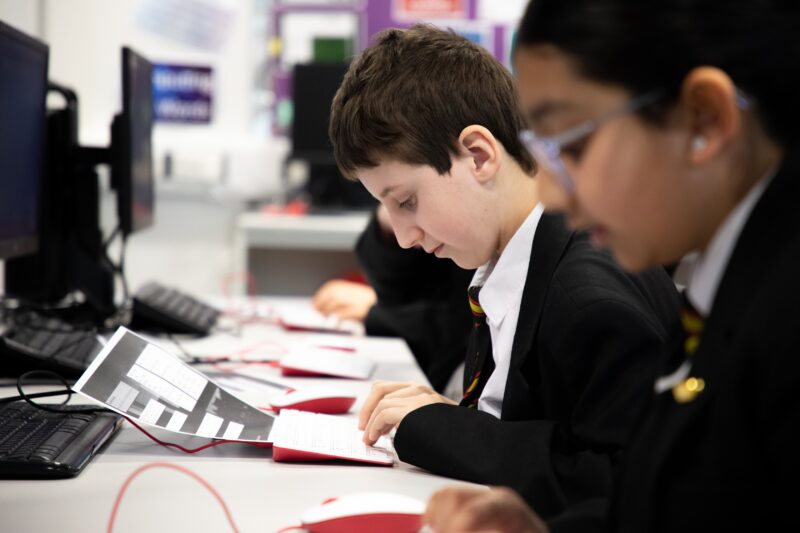
These two studies highlighted instances where PPs are more or less relevant depending on a student’s level of expertise or self-efficacy. In addition, the best PP to solve may differ from one student to another, and so having the same PP for all students to solve may be a limitation. This prompted the team to conduct their most recent study to ask how large language models (LLMs) can be leveraged to support students in code-writing practice without hindering their learning.
Generating personalised PPs using AI tools
This recent third study focused on the development of CodeTailor, a tool that uses LLMs to generate and evaluate code solutions before generating personalised PPs to scaffold students writing code. Students are encouraged to engage actively with solving problems as, unlike other AI-assisted coding tools that merely output a correct code correct solution, students must actively construct solutions using personalised PPs. The researchers were interested in whether CodeTailor could better support students to actively engage in code-writing.
In a study with 18 undergraduate students, they found that CodeTailor could generate correct solutions based on students’ incorrect code. The CodeTailor-generated solutions were more closely aligned with students’ incorrect code than common previous student solutions were. The researchers also found that most participants (88%) preferred CodeTailor to other AI-assisted coding tools when engaging with code-writing tasks. As the correct solution in CodeTailor is generated based on individual students’ existing strategy, this boosted students’ confidence in their current ideas and progress during their practice. However, some students still reported challenges around solution comprehension, potentially due to CodeTailor not providing sufficient explanation for the details in the individual code blocks of the solution to the PP. The researchers argue that text explanations could help students fully understand a program’s components, objectives, and structure.
In future studies, the team is keen to evaluate a design of CodeTailor that generates multiple levels of natural language explanations, i.e. provides personalised explanations accompanying the PPs. They also aim to investigate the use of LLM-based AI tools to generate a self-reflection question structure that students can fill in to extend their reasoning about the solution to the PP.
Barbara and Xinying’s seminar is available to watch here:
Find examples of PPs embedded in free interactive ebooks that Barbara and her team have developed over the years, including CSAwesome and Python for Everybody. You can also read more about the CodeTailor platform in Barbara and Xinying’s paper.
Join our next seminar
The focus of our ongoing seminar series is on teaching programming with or without AI.
For our next seminar on Tuesday 12 March at 17:00–18:30 GMT, we’re joined by Yash Tadimalla and Prof. Mary Lou Maher (University of North Carolina at Charlotte). The two of them will share further insights into the impact of AI tools on the student experience in programming courses. To take part in the seminar, click the button below to sign up, and we will send you information about joining. We hope to see you there.
The schedule of our upcoming seminars is online. You can catch up on past seminars on our previous seminars and recordings page.
Website: LINK